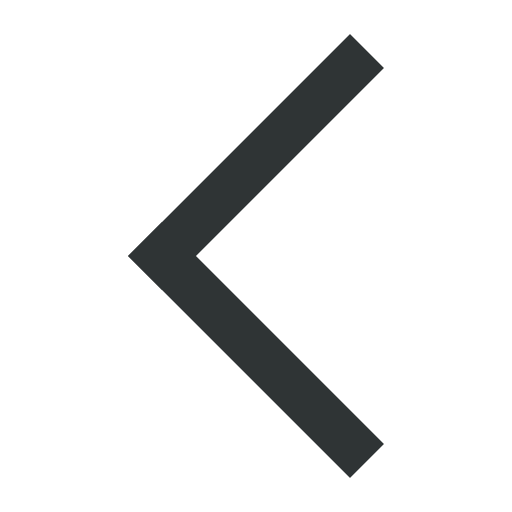
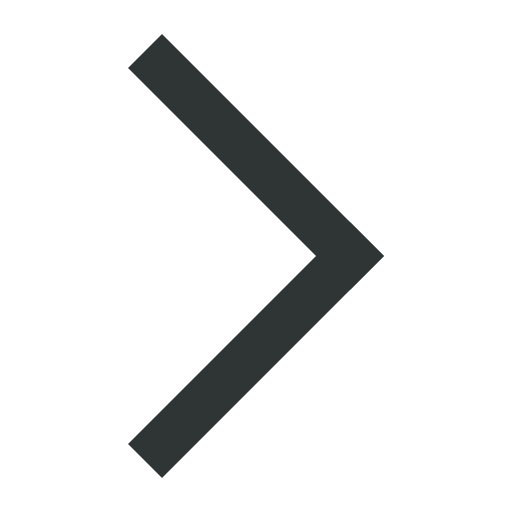

Feature article
The protein folding problem is solved. But the debate continues
![Thumbnail [Thumbnail]](https://www.iucr.org/__data/assets/image/0017/154250/thumbnail.png)
I have had two earlier articles in the IUCr Newsletter on this important research topic, the protein folding problem, including its long history (Helliwell, 2020, 2021). Firstly, I described the "everyone agreed breakthrough," especially endorsed by the judges at the Critical Assessment of Techniques for Protein Structure Prediction's 14th iteration "CASP14" of the DeepMind's entry, AlphaFold. Secondly, I described the sudden, indeed breathtaking, announcement (Cusack et al., 2021) of the collaboration of DeepMind with the EMBL EBI (European Molecular Biology European Bioinformatics Institute), whereby hundreds of thousands of predicted three-dimensional structures across 20 species were made available to the structural biology community. This gave me the chance to evaluate for myself, with one of my favourite protein crystal structures, the enzyme from sheep liver 6-phosphogluconate dehydrogenase, just how well AlphaFold2 performed. The sheep enzyme was not included in the species of the 20 provided, so instead I downloaded the three-dimensional structure for the human 6-phosphogluconate dehydrogenase amino acid sequence. It is a favourite because it was the subject of my DPhil project at the University of Oxford from 1974 to 1977 (Adams, Helliwell & Bugg, 1977). I refined the AlphaFold-predicted structure against the 2.0 Å X-ray diffraction data 2pgd (Phillips, Gover & Adams, 1995); the X-ray study had moved on from the 6 Å and 2.6 Å studies of my thesis. The difference electron-density map clearly showed a sulfate ion, which I showed as evidence to the readers of my article.
Not long after my article came out, I received the University of Washington Robetta fold prediction (https://robetta.bakerlab.org/; Baek et al., 2021) for the sheep liver 6-phosphogluconate dehydrogenase amino acid sequence that I had submitted. This did not have as good model refinement statistics or electron-density fit, but the changes needed to the predicted structure were clear from the difference map. I duly contacted Mike Glazer, as Editor, on whether an update should be provided, but we decided that the story was already clear enough. The prediction method worked, or, I can say now, both methods, i.e. DeepMind and Robetta, worked. I concluded, on this evidence, that I could have saved about a year of my DPhil time spent on solving the protein crystallographic phase problem by using these predicted structures. There were caveats though:
-
They were predictions, which Tom Terwilliger, a former Chair of the IUCr Commission on Biological Macromolecules, described in a recent virtual lecture entitled "AlphaFold changes everything: incorporating predicted models in X-ray and Cryo-EM structure determination" hosted by the University of Strasbourg, as "hypotheses".
-
It was oft-repeated that these were accurate structures, sometimes by DeepMind itself, but I hung onto their correct way of describing their structures as needing rigorous assessment via experimental data. To their credit, they had also assigned a confidence estimate to each portion of their predicted protein structure.
-
I emphasised that they are not predicting the multiple conformations of the functional states of proteins, i.e. their structural dynamics.
-
They were not predicting ligands or bound metals.
-
They were not predicting complexes of more than one protein subunit.
Progress is being made by different research teams with the fifth and fourth caveats (Perrakis & Sixma, 2021).
Skip forward a year. Accolades are being presented to the DeepMind Team based on their work (Jumper et al., 2021), such as that to the Team Leader, John Jumper, in Nature's Top Ten Researchers of the Past Year 2021 (Nature, 2021). A month ago, an article appeared with the startling title "Structural biology is solved — now what?" (Ourmazd et al., 2022). This was clearly a stunning commendation of the successes of structure prediction; but this was too much I thought. If not happy with their title, I was quite happy, however, with the reasons for explaining the breakthrough of predicting protein folds from amino acid sequences: "This delightful success is the culmination of four decades-long efforts: (1) deposition of more than 170,000 experimentally determined protein structures in the openly accessible Protein Databank; (2) deposition of a large number of amino acid sequences of entire families of proteins and their evolutionary relationships in public repositories; (3) elucidation of multiple sequence alignments; and (4) the resurgence of neural-inspired machine-learning algorithms". This last point illustrates that a new scientific method has indeed reached maturity based on big data; a detailed dissection of the methods used by Jumper et al. (2021) is given by Bouatta et al. (2021). It is not a hypothesis-driven scientific method. It is also not based on physics and chemistry, which would, as was used for many years, involve setting up force-field equations and arrive at energy-minimised, predicted, protein structures.
Ourmazd et al. (2022) went on though to state, abruptly and controversially, I would say: "Does this success mean that structural biology, as an experimental discipline, is 'solved'? Can we, in good conscience, continue to ask our students and young collaborators to spend months, if not years, determining protein structures? Or is the heyday of protein structure determination finally over? As with any success, it is important to ask what is next. We venture to believe that the full impact of structural biology is yet to come. For, as impressive as these new algorithms are, they cannot predict both protein function and mechanism directly from the amino acid sequence." My reaction had been that I could be comfortable with a predicted protein structure, the hypothesis of Tom Terwilliger, if I could refine it against my X-ray diffraction data observations. It would be a game-changer if the model refinement stage were to arrive much more expeditiously than if I had to first solve the protein crystallographic phase problem. Metal content, if any, would be determined by X-ray fluorescence and the identity of the metal atoms and their positions determined by crystallography with tunable X-ray wavelength resonant scattering signals. Ionisable amino acid protonation states would be determined by neutron protein crystallography. Then I would embark on the structure and function stage of research via time-resolved diffraction studies in the crystal or in solution or an ensemble of static crystal structures of a protein, and cryoEM ensembles. The study of the structure and function of 6-phosphogluconate dehydrogenase continues to this day, for example by Hanau & Helliwell (2022).
These observations clearly show that Ourmazd et al. (2022) had drawn the boundary between prediction and experiment incorrectly. Terwilliger's recent lecture slide 27 (Fig. 1) shows the likely workflow for protein crystallography in the future, with which I obviously concur (note his starting point at the top left with the AlphaFold prediction for each protein chain).
![[Fig1]](https://www.iucr.org/__data/assets/image/0020/154316/Fig1.jpg)
A few weeks later, on 4 February 2022, a new Viewpoint appeared in Science (Moore et al., 2022), not citing the Ourmazd et al. (2022) article but taking the opposite end of the spectrum of views in their article titled "The protein-folding problem: Not yet solved". This article includes the assertion that "At present, for the best cases, the C-alpha coordinate RMSD accuracy of AlphaFold-predicted structures roughly corresponds to the accuracy expected for structures determined at resolutions no better than ~4 Å." Furthermore, they state that "(we) feel that solving the protein-folding problem means making accurate predictions of structures from amino acid sequences starting from first principles based on the underlying physics and chemistry."
A point on which we all agree though is well put by Moore et al. (2022), who state, "A further complication for structure prediction is the dynamic structural variation in a given sequence."
What about the diffraction resolution for which the AlphaFold model can be trusted then? 2 Å or only 4 Å? Taking the sheep liver 2pgd X-ray diffraction data to 2 Å resolution and the human 6-phosphogluconate dehydrogenase predicted structure, the restrained model refinement converges quite normally to an R factor of 21%, which is indeed typical. Moreover, the R factor versus resolution is shown in Fig. 2 and again is typical.
![[Fig2]](https://www.iucr.org/__data/assets/image/0003/154317/Fig2.jpg)
Also note that this set of model refinement statistics is for a model with no bound waters or ions, which AlphaFold of course does not predict, and which would improve the R factor further. In terms of electron-density maps, Fig. 3 shows the 2pgd model and the AlphaFold-predicted structure after model refinement against the 2pgd X-ray diffraction data. The sulfate position and bound waters are placeable without difficulty in this 2 Å high-resolution electron-density map.
![[Fig. 3]](https://www.iucr.org/__data/assets/image/0008/154484/coot_2pgd_Xray_AlphaFold-modela.png)
In summary, AlphaFold and Robetta will boost the solving of experimental structures by X-rays and electrons. Their models will help those researchers struggling with electron density or electrostatic potential map interpretations of their polypeptides. However, only when we have included in these prediction algorithms the real (or close to real) forcefields, can we really think about having solved the biological structure and dynamics challenges. Nevertheless, the function prediction may well still be left behind unless determined action by funding agencies is taken, which is Ourmazd et al.’s main point. Also, nothing is definitively solved, a lot is still to come and to learn, which is Moore et al.’s main point.
Acknowledgement
I am very grateful to Adriana Miele, Full Professor of Biochemistry and Biophysics of the University of Lyon, for discussions and her stimulus to attempt a conclusions paragraph.
References
Helliwell, J. R. (2020). DeepMind and CASP14. IUCr Newsl. 28(4).
Helliwell, J. R. (2021). Reaction to announcement of AlphaFold Database. IUCr Newsl. 29(2).
Perrakis, A. & Sixma, T. K. (2021). AI revolutions in biology. EMBO Rep. 22, e54046.
John R. Helliwell is Emeritus Professor, Department of Chemistry, University of Manchester, UK, and is a DSc in physics from the University of York, UK; john.helliwell@manchester.ac.uk.
Copyright © - All Rights Reserved - International Union of Crystallography