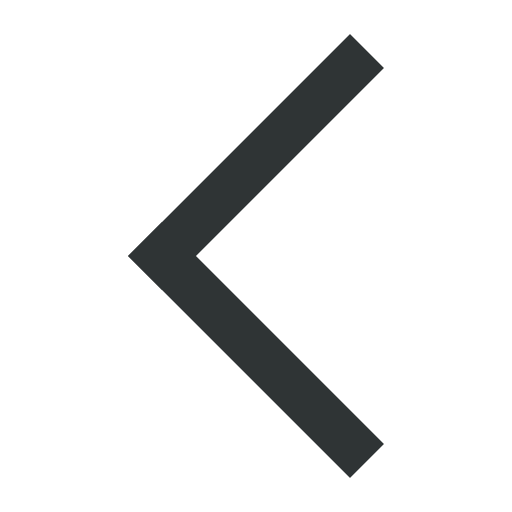
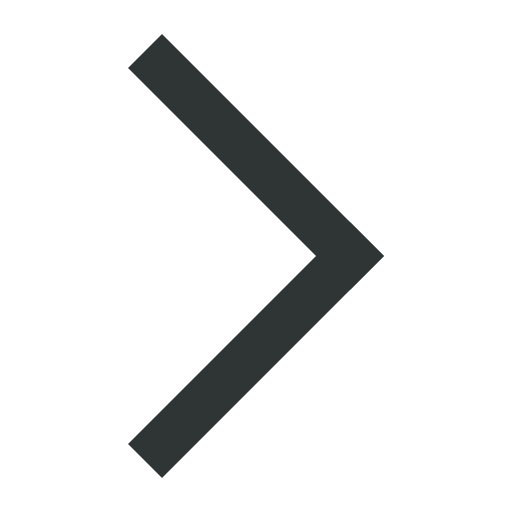
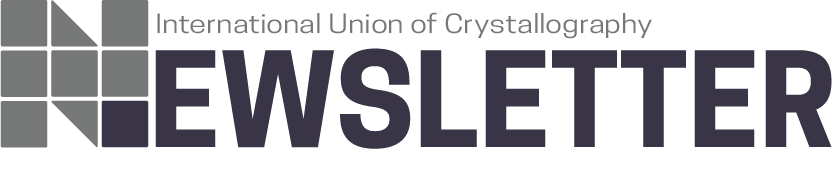
Feature article
Machine learning in crystallography
![Thumbnail [Thumbnail]](https://www.iucr.org/__data/assets/image/0007/154249/TOC-Image-ML_journalcolours.png)
Figure 1. Number of articles matching the search term 'machine learning' by IUCr journal. Data compiled from site https://journals.iucr.org/ (15 February 2022).
By now, everyone has probably heard the term 'machine learning'. We have all used tools in our everyday lives that use machine learning, from voice assistance to image recognition to ever more sophisticated algorithms showing us online ads of what we supposedly want to buy. There have been great advances in medicine based on machine learning, especially in the area of radiology. As more of our lives are affected by this new technology, the question of bias and the limits of its application have come up – an interesting read on these aspects is Cathy O'Neil’s book Weapons of Math Destruction [1].
The question in many researchers' minds is what is the impact of machine learning and artificial intelligence (AI) going to be on their field of science – in this case, crystallography. A quick full-text search of the term 'machine learning' in all IUCr journals reveals 296 articles (as of February 2022). While this is clearly a non-comprehensive survey of crystallographic papers using machine learning in some form, and this simple full-text search has its limitations, a quick breakdown by journal (Fig. 1) gives an interesting perspective on the breadth of areas within crystallography on which machine learning has an impact.
Interestingly, the first paper in this search was published in 1993 in Acta Cryst. D on integrating direct methods and AI strategies for solving protein structures [2]. Structural biology has remained a field active in exploring machine learning and AI in order to move forward. However, across nearly all the journals and the related fields, articles exploring how machine learning and related techniques can be applied go back 20+ years. Browsing through the list of papers was an excellent way to bring up ideas from work in a completely different context and made me appreciate the breadth of crystallography as a discipline.
One approach is so-called supervised machine learning, where labelled data are used to train a neural network that is then used for classification or regression of other data. Image recognition is a prime example. Once you train a neural network with enough labelled images (i.e. the computer gets told what is on the image) of cats and dogs, the network will be able to identify cats and dogs on other images. Because we can often calculate the signal we expect from an experiment based on a model, we can create training data through simulation and use the model parameters as labels. This allows a speed up of expensive calculations by having the neural network ‘interpolate’, but it can also be used to, for example, determine the space group from diffraction and pair distribution function data [3, 4]. This method of classification also opens the door to machine-learning-generated metadata, for example to label experimental data as 'good', 'bad', 'misaligned' and so on. While these labels might need verification by a scientist, this approach offers an easy way of flagging problems, especially on high-throughput instruments.
One of the recent exciting developments is that machine learning has become much more accessible, thanks to advanced and easy-to-use tools developed by the major tech companies. This gives us access to machine-learning libraries such as TensorFlow [5] or PyTorch [6] and easy-to-use tools requiring virtually no coding. I am involved in Oak Ridge Computer Science Girls [7], and we teach middle-school girls about machine learning using Teachable Machine [8]. Here, they can train a neutron network to classify images. The impact of these tools became evident in a session on AI at the 2021 ACA meeting, when Scott Classen from the Advanced Light Source Berkeley presented machine learning aiding sample centering and included a 'behind the scenes' look of how the tool was built using essentially a Google Cloud Drag and Drop tool [for details see 9]. In the same session, Dan Olds, from Brookhaven National Laboratory, presented an in-depth overview of the many areas in which machine learning and AI will have an impact on user facilities in accelerating scientific discovery and making using a beamline more streamlined. He introduced a novel approach to use so-called reinforcement learning to have the system 'learn' what a good experiment looks like [10].
In summary, there is a lot of exciting work involving machine learning in the crystallographic community from structure solution to data analysis and running smarter experiments. In many ways, this is just the start. So will machine learning replace crystallographers? No, but crystallographers using machine-learning techniques will likely surpass those who do not.
References
Copyright © - All Rights Reserved - International Union of Crystallography